Key Takeaways
- Diverse AI Models: Artificial intelligence models can be categorized into supervised, unsupervised, and reinforcement learning, each serving unique purposes and applications across various industries.
- Crucial Technologies: Key technologies like machine learning, deep learning, and natural language processing form the foundation of AI models, enabling them to process data and make predictions effectively.
- Industry Applications: AI models are transforming sectors such as healthcare, finance, and transportation, driving innovation through enhanced decision-making, predictive analytics, and operational efficiencies.
- Challenges and Solutions: Developing AI models comes with challenges like data privacy concerns and model bias, necessitating strategies such as data anonymization and bias mitigation to foster ethical AI systems.
- Emerging Trends: Future advancements in AI are focused on automated machine learning, explainable AI, and federated learning, emphasizing efficiency, transparency, and data privacy in applications.
- Inclusive and Adaptive Design: The trend towards neuro-inclusive AI and self-supervised learning underscores the importance of creating accessible, efficient models that cater to diverse learning styles and reduce reliance on labeled data.
Artificial intelligence models are transforming the way we interact with technology, revolutionizing industries from healthcare to finance. These sophisticated systems analyze vast amounts of data, learning patterns and making predictions that were once unimaginable. As AI continues to evolve, understanding its underlying models becomes crucial for leveraging its full potential.
In an age where data drives decision-making, the ability to harness AI models can give businesses a competitive edge. From natural language processing to computer vision, these models are not just buzzwords; they’re the backbone of innovative solutions that enhance efficiency and productivity. As we delve deeper into the world of artificial intelligence, the importance of these models only grows, shaping the future of technology and society.
Artificial Intelligence Models
Artificial intelligence models represent complex systems designed to process and analyze data, uncover patterns, and facilitate decision-making. These models range from simple algorithms to advanced neural networks, each serving unique purposes across various sectors.
Types of Artificial Intelligence Models
- Supervised Learning Models
Supervised learning models operate on labeled data sets to identify relationships between input variables and output results. These models aim for high accuracy in predicting outcomes, commonly used in applications like image recognition and spam detection.
- Unsupervised Learning Models
Unsupervised learning models analyze unlabeled data to detect hidden structures or groupings. They excel in clustering and association tasks, useful in market segmentation and social network analysis.
- Reinforcement Learning Models
Reinforcement learning models learn optimal actions through trial and error in dynamic environments. They effectively optimize decision-making processes in applications such as robotics and game playing.
Key Technologies Behind AI Models
- Machine Learning
Machine learning forms the backbone of many AI models, utilizing algorithms to parse data, learn from it, and make informed predictions or decisions without explicit programming.
- Deep Learning
Deep learning employs neural networks with several layers to automatically learn hierarchical representations of data. This technology often handles complex tasks, including natural language processing and autonomous driving.
- Natural Language Processing
Natural language processing (NLP) enables machines to understand and respond to human languages, enhancing interactions in customer service chatbots and virtual assistants.
Applications of Artificial Intelligence Models
Artificial intelligence models find applications across diverse industries, including:
- Healthcare: Predicting disease outbreaks, diagnosing conditions, and personalizing treatment plans.
- Finance: Fraud detection, risk assessment, and algorithmic trading strategies.
- Retail: Inventory management, customer behavior analysis, and personalized marketing.
Understanding these artificial intelligence models empowers businesses to leverage technology effectively, boosting operational efficiency and driving innovation.
Types of Artificial Intelligence Models
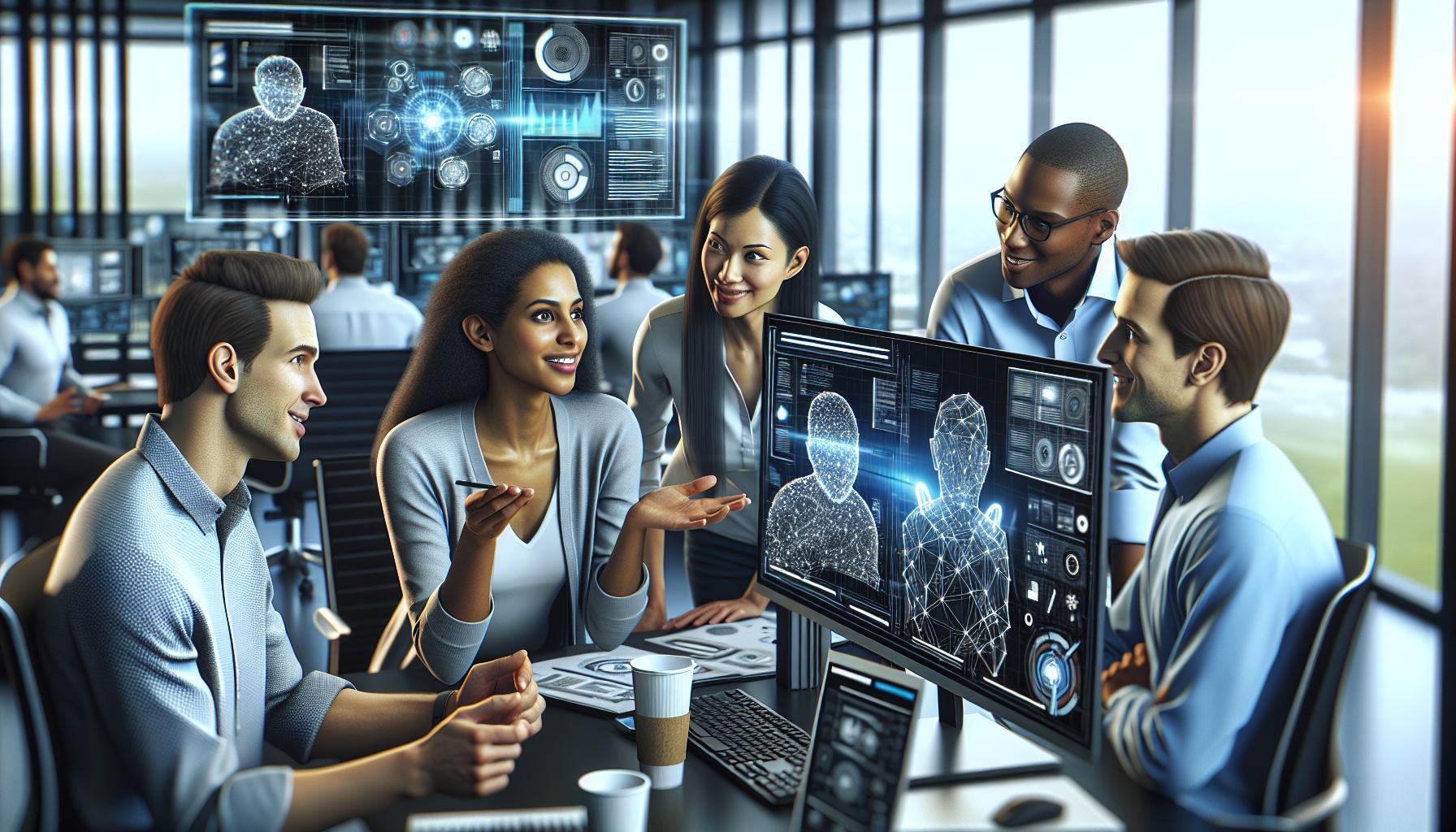
Artificial intelligence models fall into distinct categories based on their learning approaches. Each type serves specific functions and applies varied methodologies to process data efficiently.
Supervised Learning Models
Supervised learning models utilize labeled data for training purposes. These models rely on input-output pairs to develop predictive algorithms. Common applications include image recognition, spam detection, and credit scoring. Models like linear regression, decision trees, and support vector machines are prevalent in this category. This approach requires a substantial amount of annotated data, enhancing predictive accuracy and reliability.
Unsupervised Learning Models
Unsupervised learning models analyze unlabeled data to uncover patterns and relationships. These models extract insights without pre-existing classifications. Typical applications comprise customer segmentation, market basket analysis, and anomaly detection. Common algorithms include k-means clustering, hierarchical clustering, and principal component analysis (PCA). This flexibility allows businesses to identify hidden structures within datasets, driving strategic decision-making.
Reinforcement Learning Models
Reinforcement learning models optimize decision-making through trial and error. These models learn optimal behaviors by receiving rewards or penalties based on their actions in a given environment. Applications include game playing, robotics, and automated trading systems. Notable approaches in this area include Q-learning and deep reinforcement learning. This model fosters proactive learning, enabling systems to adapt and improve over time through experience.
Applications of Artificial Intelligence Models
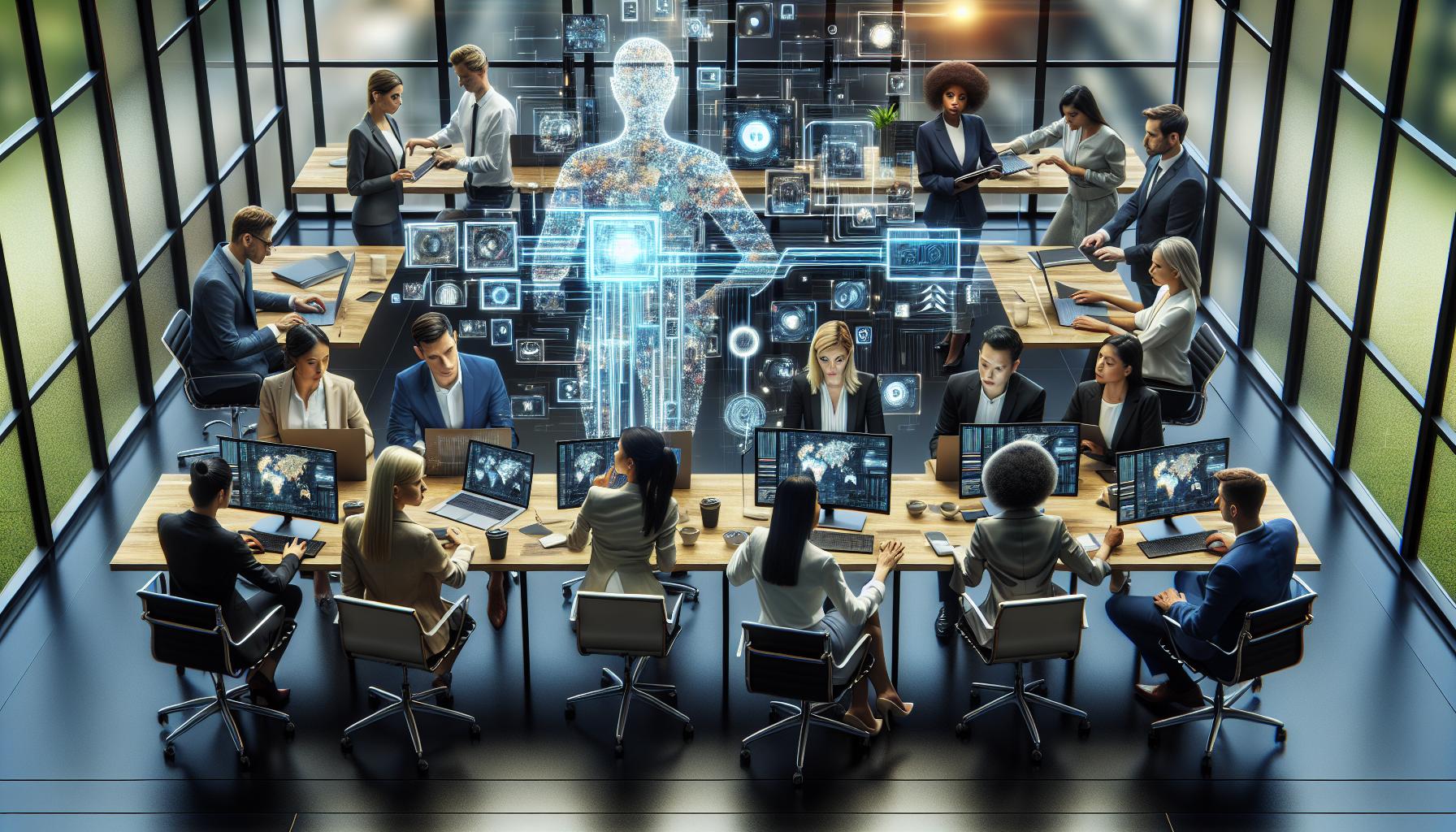
Artificial intelligence models play a crucial role in transforming various industries. They enable enhanced decision-making, predictive analytics, and operational efficiencies across sectors.
Healthcare
AI models improve patient outcomes by predicting disease progression and optimizing treatment plans. They analyze medical data to identify patterns in patient symptoms, leading to early diagnosis and personalized healthcare. For instance, AI algorithms assist radiologists by accurately detecting tumors in imaging scans, reducing diagnostic errors. Furthermore, predictive analytics helps anticipate patient admissions, allowing for better resource allocation in hospitals.
Finance
In the finance sector, AI models streamline operations and reduce risks. They assess credit risk by evaluating an applicant’s financial history and behavior patterns, enhancing loan approval processes. AI models also detect fraudulent transactions by identifying unusual spending behaviors in real-time. Additionally, algorithmic trading systems utilize AI to analyze market trends and execute trades at optimal times, maximizing profits while minimizing losses.
Transportation
AI models enhance transportation efficiency through route optimization and predictive maintenance. They analyze traffic patterns and weather data to provide real-time navigation guidance, thereby reducing travel times. Autonomous vehicles rely on AI models to interpret sensor data and make driving decisions, improving safety. Predictive maintenance models also monitor vehicle conditions, alerting operators to potential issues before they lead to breakdowns, thereby minimizing downtime.
Challenges in Developing Artificial Intelligence Models
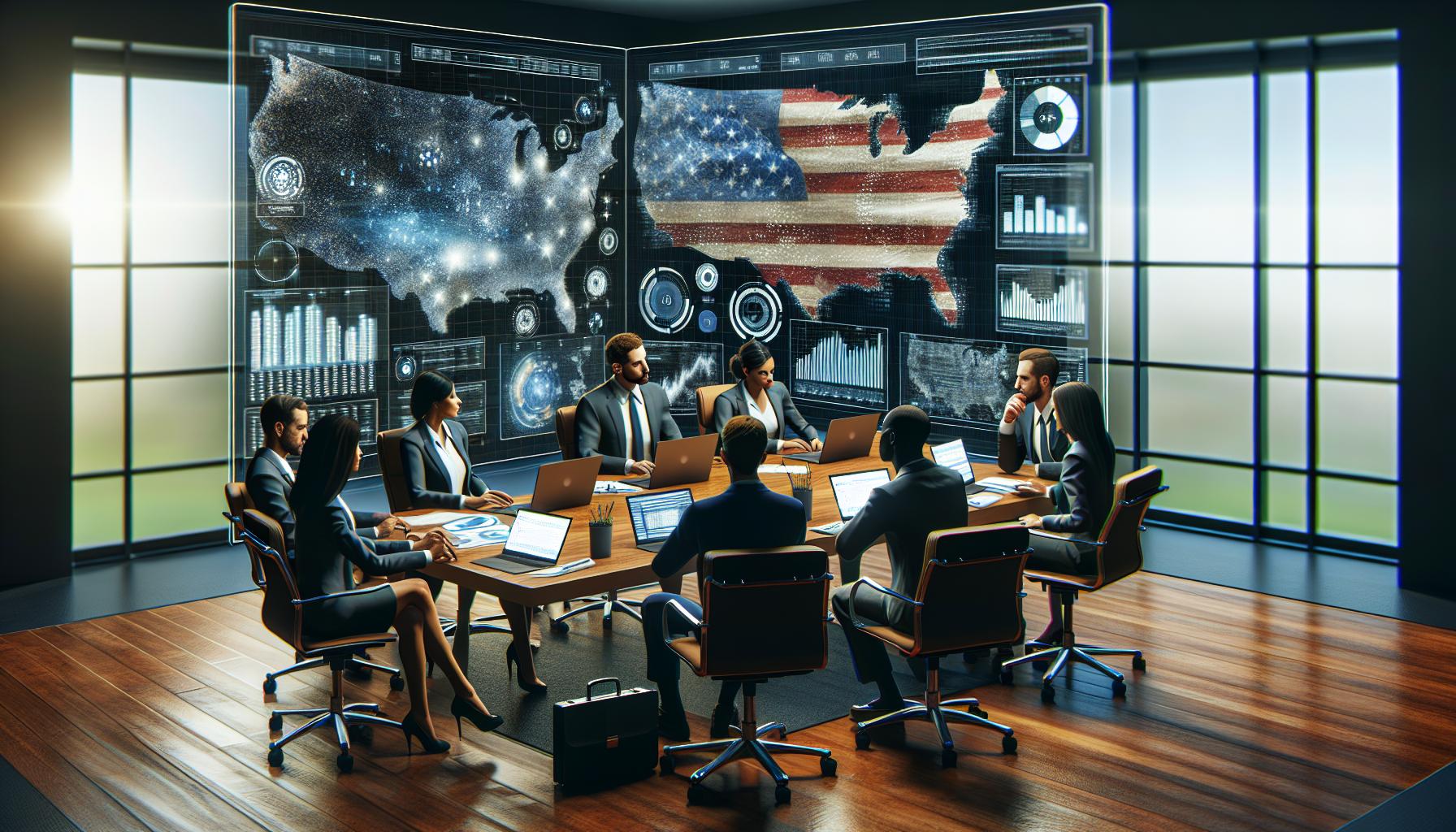
Developing artificial intelligence models entails various challenges that can impact their effectiveness and acceptance. Addressing these obstacles is crucial for creating robust, reliable, and ethical AI systems.
Data Privacy Concerns
Data privacy issues pose significant challenges for AI model development. Organizations rely on large datasets that often contain sensitive information. Failure to protect this data can lead to breaches that compromise user privacy and legal non-compliance. Adhering to regulations like the GDPR mandates transparency and consent from users regarding data usage. Implementing anonymization techniques helps mitigate risks, allowing for meaningful analysis without compromising individual privacy.
Model Bias and Fairness
Model bias affects the performance and fairness of AI systems. If training data reflects historical prejudices or imbalances, the model may produce biased outcomes, negatively impacting certain demographic groups. Ensuring fairness involves diversifying training datasets and employing algorithms that detect and mitigate bias. Regular audits and assessments of AI systems help identify disparity in predictions, fostering inclusivity and equitable treatment across applications.
Future Trends in Artificial Intelligence Models
Future trends in artificial intelligence models indicate significant advancements in technology and application across various sectors. Emerging developments focus on enhancing model efficiency, minimizing bias, and improving interpretability.
- Automated Machine Learning (AutoML)
Automated machine learning simplifies the process of model selection and hyperparameter tuning. Users can generate high-performance models with minimal expertise, democratizing access to AI capabilities. This trend accelerates deployment in industries lacking extensive data science resources.
- Explainable AI (XAI)
Explainable AI emphasizes the need for interpretability in complex AI models. Organizations seek transparency in decision-making processes, particularly in critical areas like healthcare and finance. XAI technologies aim to bridge the gap between model accuracy and user understanding.
- Federated Learning
Federated learning enables decentralized model training across multiple devices while preserving data privacy. This approach allows organizations to utilize large datasets without compromising sensitive information, adhering to regulations like GDPR. Adoption increases among industries reliant on confidential data.
- AI Ethics and Governance
As AI becomes integral to decision-making, ethical considerations take center stage. Organizations prioritize ethical frameworks to mitigate bias and ensure fair outcomes. This trend nurtures public trust and promotes responsible AI use across sectors.
- Neuro-Inclusive AI
Neuro-inclusive AI focuses on catering to diverse cognitive profiles. Developers create models that accommodate various learning styles, enhancing accessibility and user experience. This trend fosters inclusivity in AI application design, expanding user engagement.
- Self-supervised Learning
Self-supervised learning minimizes the reliance on labeled datasets, allowing models to learn from unstructured data. This method increases data efficiency and expands learning opportunities, significantly impacting image and natural language processing tasks.
- Hybrid AI Models
Hybrid models integrate different AI methodologies, such as combining symbolic reasoning with machine learning. This approach leverages the strengths of each technique, yielding more robust solutions capable of handling complex tasks with improved performance.
These trends signify a future where artificial intelligence models evolve to maximize efficiency and ethical responsibility. As businesses adopt these advancements, they create opportunities for innovative applications across sectors while addressing potential challenges associated with model development and deployment.
Artificial Intelligence Models
Artificial intelligence models are revolutionizing how industries operate by enhancing decision-making and operational efficiency. As businesses continue to embrace these technologies, understanding their complexities becomes essential for maximizing their potential.
The future promises even more innovations with advancements like Automated Machine Learning and Explainable AI, which aim to simplify processes and increase transparency.
Navigating the challenges of data privacy and bias will be crucial for organizations striving to implement AI responsibly. By prioritizing ethical considerations and inclusivity, companies can harness the full power of AI models, driving progress and fostering a more equitable technological landscape.